Our Investment strategy
Scientific approach, advanced technology, and experience protecting your investments even during crisis
Expected indicators of the strategy
Annual Return
Maximum Drawdown over 20+ years, Market -55% in 2008
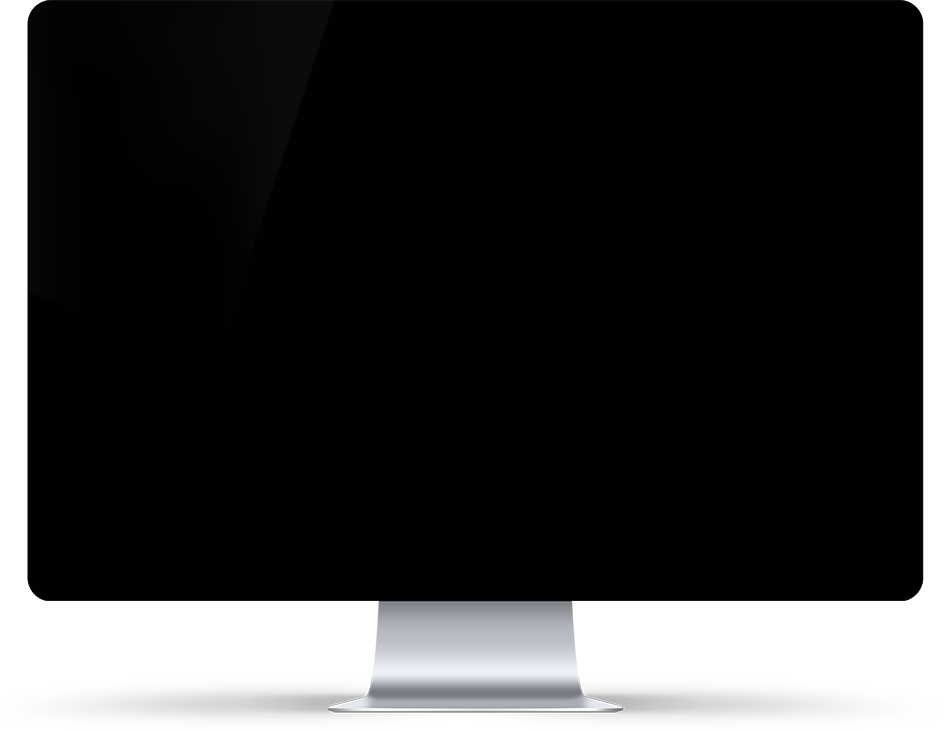
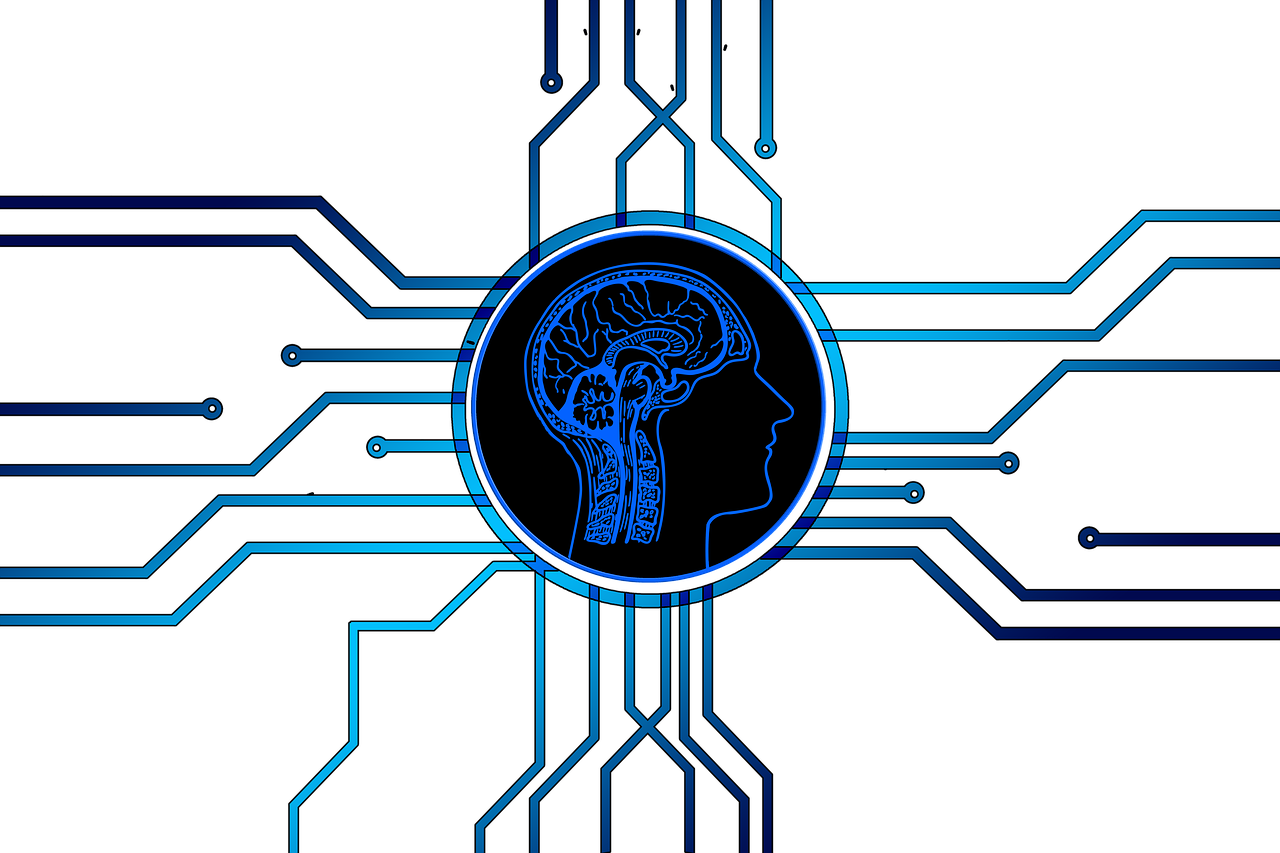
What do we do?
We are a quantitative investment management company investing in developed market equities around the globe.
- Quantitative:Using advanced statistical and machine learning models.
- Systematic:100% following models.
- Efficient:Cost-effective execution of trades.
- Risk return tradeoff:Balancing returns and risks taken.
Our investment universe
We invest into publicly traded stocks from developed markets, including U.S., Canada, Europe, Japan, Australia, etc.
The investment universe as broad as ours offers a greater number of mispriced investing opportunities as well as stronger diversification relative to other regionally restricted strategies, and relative to other asset classes.
Our exposures to individual countries and regions varies over time and is fully dictated by our model.
Regional decomposition of our investment universe can be seen on the right.
Machine learning methodology
We use ensembles of state-of-the-art machine learning models:
- Neural Networks
- Gradient Boosted Regression Trees
- Random Forests
... in multiple prediction tasks:
- Excess stock returns
- Rank of the stock returns
- Classification of extreme stock returns
... tested in the rigorous statistical setting.
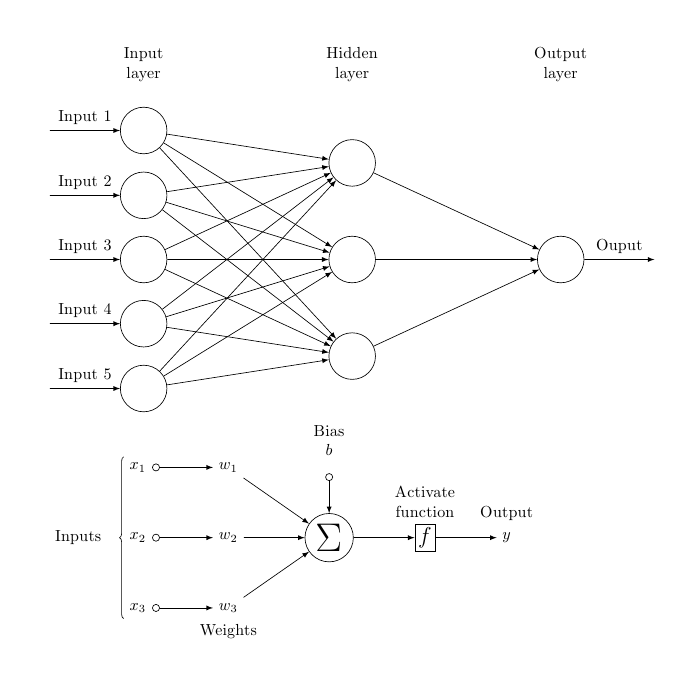
Weight of the evidence
Expectations based on the evidence from the large-scale data on equities from around the globe going back to 1926.
More than 50 000 firms from (details see on the chart in the right):
- North America (U.S., Canada)
- Europe (Austria, Belgium, Denmark, Finland, France, Germany, Greece, Ireland, Italy, Luxembourg, the Netherlands, Norway, Portugal, Spain, Sweden, Switzerland, and the United Kingdom)
- Japan
- Asia Pacific (Australia, New Zealand, India, and China)
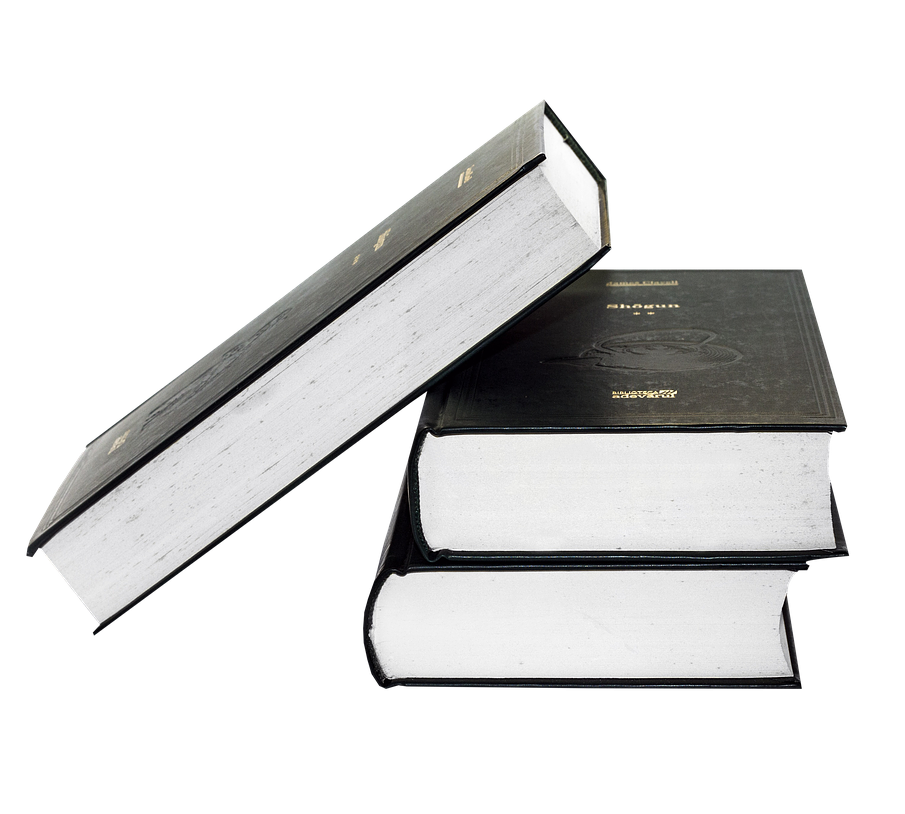
Academic papers we read
We continuously monitor academic research and closely cooperate with researchers from universities.
Peek into our library:
- Lewellen, Jonathan, Stefan Nagel, and Jay Shanken. "A skeptical appraisal of asset pricing tests." Journal of Financial economics 96, no. 2 (2010): 175-194.
- DeMiguel, Victor, Lorenzo Garlappi, and Raman Uppal. "Optimal versus naive diversification: How inefficient is the 1/N portfolio strategy?." The review of Financial studies 22, no. 5 (2007): 1915-1953.
- Srivastava, Nitish, Geoffrey Hinton, Alex Krizhevsky, Ilya Sutskever, and Ruslan Salakhutdinov. "Dropout: a simple way to prevent neural networks from overfitting." The journal of machine learning research 15, no. 1 (2014): 1929-1958.
We believe that picking stocks is no easier than telling which of the stars above will shine more in three years. Hence observations, data, and technology are a must.
Without data and technology, you are lost.
Technology stack
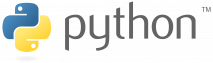
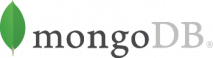
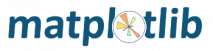
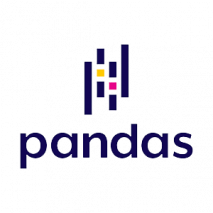
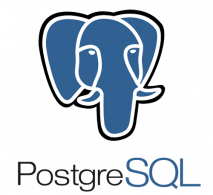
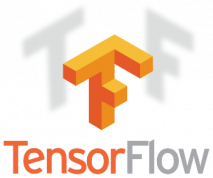
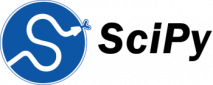
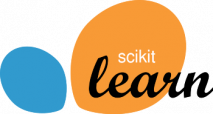
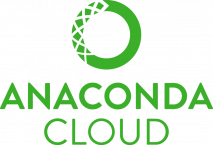
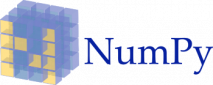